Artificial Intelligence (A.I) is set to Revolutionize Biology!
For decades, biology has been plagued by an unresolved enigma that has slowed medical development. However, this does not negate the reality that we have made significant advances in science and technology over time. The Protein-Folding problem has been a major source of frustration for biochemists and drug designers attempting to understand and save lives.
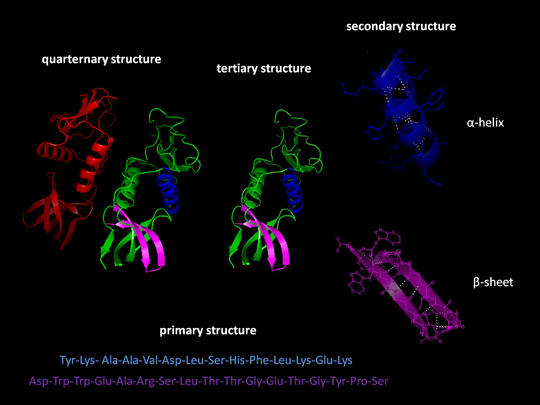
Proteins are vital for survival, yet predicting their appearance is extremely difficult. I however believe that "There is always a solution to every problem," and this was the case a few months ago when two independent groups declared to an enthusiastic scientific community that they had conquered the menace, thanks to some well-crafted artificial intelligence. I believe this is incredible, as it could lead to advancements in the fight against cancer, COVID-19, and even hazardous waste! The possibilities seem endless!
Proteins make up everything in our bodies and all other species, including viruses, serving as the building blocks of life. They have a role in the transport of oxygen in our blood, food digestion, DNA replication, infection resistance, structure formation, and much more. They're way more than just foods that help us build muscle and tissues. To elaborate, DNA (which is also the code that makes you who you are) is essentially a set of instructions for producing these proteins.
Proteins are made up of a total of 20 molecules known as amino acids, which are the basic building blocks of proteins. Try visualizing proteins as words and amino acids as the letters that make up those words! The reading of DNA instructions to build lengthy strings of amino acids that fold in on themselves in specific ways and settle into specific shapes is brief description of the process by which proteins are produced in the body. Proteins must fit together like puzzle pieces or bind to specific molecules, so their shapes determine how they function.
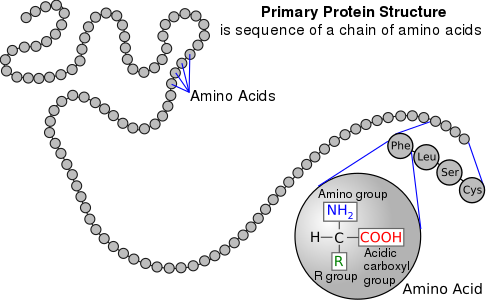
Sourced from National Human Genome Research Institute
This distinguishes Proteins from DNA, where knowing the sequence of that DNA tells you what it does. Shape is utmostly crucial when it comes to proteins. The sequence determines how an amino acid string folds, and if it can be deciphered, a researcher should be able to determine the final shape or shapes of that particular protein. It's relatively simple to figure out what the sequence should be because it's derived from DNA and a researcher can read the genetic code. Since a protein can contain anywhere from fifty to two thousand amino acids, the latter part of working out the shape is much more difficult. Do have it in mind that each amino acid has a slightly different chemical structure which makes it only more complex.
Individual amino acid parts can interact with all other amino acids in close proximity, as well as certain amino acids from afar, setting the folding in seemingly random directions. And yes, we have a treasure trove of databases containing the amino acid sequences of billions of proteins, but scientists have always been stuck in the dark about their actual shapes.
Scientists use X-ray Crystallography, which involves slowly evaporating a solution containing proteins until the proteins left behind form crystals. X-ray beams are then fired at the crystals to get images that are assembled into a 3D model. . Another technique for determining the structure of proteins is NMR imaging (Nuclear Magnetic Resonance), which is similar to the technology used in hospitals to image the human body. Both methods take a long time to complete, and depending on the protein, it could take days or even years.
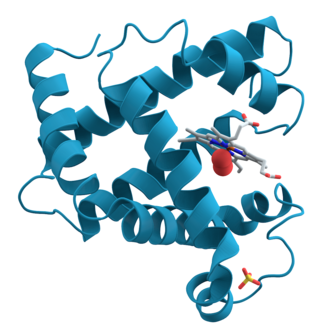
Sourced from AzaToth
Usher in the year 2020, a team from DeepMind (a Google-owned company based in London) made a stunning announcement to the world, claiming that their new A.I algorithm, "AlphaFold2" could predict the folded shape of proteins from amino acid sequences in a manner that was very similar to experimental methods. The firm is well-known for developing artificial intelligence (AI) that has defeated world champions in chess, Go, and StarCraft 2.
They competed in a competition titled CASP14 in 2020 to prove their claim was correct. The competition happens to be one in which Researchers attempting to solve the protein folding problem with computers compete every two years. Prior to 2020, no one had come close to accurately predicting the protein structures of amino acids with known sequences. Even DeepMind's first version of AlphaFold, which competed for the first time in 2018, couldn't pull it off.
Two-thirds of AlphaFold2's predictions were as good as experiments. This implies that not all predictions were accurate, as AlphaFold2 disagreed with experiments on a few occurrences. The question of how close the predictions of the protein structure are to the real structures in comparison to the experimental structures available is still being debated. This occurs due to the fact that all predictions and experiments have a margin of error.
It is also worthy to note that AlphaFold2's predictions were incredibly accurate, with margins of error as small as a typical atom in some cases! It literally could guess the exact location in the overall structure of each of the thousands of individual atoms. What more could anyone possibly ask of AlphaFold2? Scientists were astounded by the breakthrough, referring to it as a "Holy Grail" of sorts.
A group of researchers from the University of Washington in Seattle decided to apply the algorithm DeepMind created to their work on the problem, based on what they saw. They developed their own algorithm, dubbed "RoseTTAFold" in partnership with an international team of collaborators. In a couple of ways, this program outperformed AlphaFold.
Given my enthusiasm for open-source software, both the DeepMind and Seattle teams published their complete methods in July 2021, making their code available to academics all over the world. Check out their github links below before the comment section. The possibilities for how we use them are endless, and I'm excited to see what amazing things A.I. technology will facilitate. DeepMind has also published a paper with structure predictions for almost all human proteins! Isn't it tremendous?
And there's more! Since scientists are working around the clock to develop drugs that prevent proteins from binding to each other, as can be seen in our fight against cancerous cells and viruses, being able to modify and design new proteins is usually part of the process. The awesome fact that researchers can now see the proteins they're designing on a computer screen without having to go to great lengths to make them remains outstanding as t his could reduce the time the process takes to a few days rather than years!
I believe that a lot can be done with these A.Is, especially with exotic ideas for Proteins. When harmful chemicals are broken down, proteins produce useful byproducts. Hence, Artificial proteins that can break down toxic waste or produce biofuels can be designed using artificial intelligence.
-->Do you want to know how these teams of scientists were able to bring home the holy grail of biochemistry? The second half of this article will be published here on Hive in my favorite community, @Stemsocial, so keep an eye out for it in the coming days!
The article is already long enough for a good read. For the time being, feel free to share your thoughts on what you believe science's future will be as A.I continues to be integrated into our daily lives!
GitHub Links!
AlphaFold
RoseTTAFold
Wanna read up some more? Check out these links!
Protein Folding
Highly accurate protein structure prediction with AlphaFold
AlphaFold: a solution to a 50-year-old grand challenge in biology
RoseTTAFold: Accurate protein structure prediction accessible to all
Congratulations @mengene! You have completed the following achievement on the Hive blockchain and have been rewarded with new badge(s):
Your next target is to reach 150 posts.
You can view your badges on your board and compare yourself to others in the Ranking
If you no longer want to receive notifications, reply to this comment with the word
STOP
To support your work, I also upvoted your post!
Check out the last post from @hivebuzz:
Support the HiveBuzz project. Vote for our proposal!
These algos are cool but the drawbacks are not always highlighted.
Generally, these methods are good at predicting but lack when it comes to explaining the underlying dynamics because DL and ML techniques are mostly advanced pattern recognitions algos.
How 'bout an algo that is selected based on its ability to explain the inner workings of other algos to us? :D
But maybe you're not talking about neural networks.
That would be one to call a Legend! I'm sure someone working on that problem already :)
neural networks are essentially statistical models. So they find statistical patterns and cannot find causal patterns. We need a new type of algos to resolve this problem
I think what you're describing might just be impossible! David Hume said that when we observe a billiard ball hitting another ball and then the second ball starts moving, we didn't really witness a billiard ball causing another to move, all we saw was the first ball stopped and then the second started moving. We don't observe causation, we infer or invent it. We observe what Hume called 'constant conjunctions', i.e. putting one's hand in the flame is always associated with one's hand burning. So what science does, is they come up with a theory that posits (invents) causation and makes predictions, and if those predictions come true (molecules moving very fast would create heat and that would cause one's hand to burn etc.), then we say the theory is true.
Now maybe algos could do that too, but it would all be based on statistical patterns anyway! "All cases of putting one's hand in the flame so far have led to burning, therefore my statistical apparatus says fire 'causes' burning". The algo will necessarily generalize a small part of the world to the whole of the world. And as Bertrand Russell said, "The man who has fed the chicken every day throughout its life at last wrings its neck instead, showing that more refined views as to the uniformity of nature would have been useful to the chicken."
There is a subtle nuance here/ DL/ML techniques don't make the pattern explicit. They identify the statistic but don't identify the causation.
Without the causation part each topic down here https://www.tylervigen.com/spurious-correlations would be worthy of a nobel-prize :3
This somewhat relevant article from Guardian found its way to my inbox this morning.
That seems interesting I will bookmark it and give it a read later :3
Yeah sure, it's all about prediction. Seems patterns can be seen in quite a lot of things around us.
Thanks for your contribution to the STEMsocial community. Feel free to join us on discord to get to know the rest of us!
Please consider delegating to the @stemsocial account (85% of the curation rewards are returned).
You may also include @stemsocial as a beneficiary of the rewards of this post to get a stronger support.
Science, technology and neuroscience, among other branches of research, have great discoveries in store for us in the not too distant future. The new generations will be in charge of maintaining life on the planet for trillions of years to come. Thank you for sharing such interesting and educational information. Best regards @mengene ...
The future would be incredibly awesome if we continue to put science to good use! I'm honored that you find this article interesting and educational. I look forward to seeing you in the second half of the article!
This would be huge!
There's always a solution to every problem indeed, the question is how soon we'll find it!
The sooner the better... So i've been told! 😃
I'm excited at the accelerated growth in tech and the breakthroughs we continue to have!
Dear @mengene, we need your help.
Your support for our previous proposal has been much appreciated but it expired end of December!
>The Hivebuzz proposal already got an important support from the community and is close to be funded. However, it misses a few votes to get past the return proposal and your could make the difference!
May we ask you to support it so our team can continue its work this year?
You can do it on Peakd, ecency, Hive.blog or using HiveSigner.
https://peakd.com/me/proposals/199
Thank you!
Hi, @mengene your post highlights what can be done from the formality of research. Artificial Intelligence is a branch that has been growing and has given many applications answers or alternatives. Excellent, that you highlight the contribution of Artificial Intelligence to Biology. Thank you for such an enriching post.
A.I is here to stay! All fields of research will definitely have A.I integrated in lots of studies! Drug design only just got easier opening the floor to all!
I appreciate you reading through and finding value in the content! Cheers
Thanks for this interesting post.
@mathowl is very correct. In addition, those tools are good in interpolating, but who knows what they can do when it turns to extrapolating. This is to me one of the most important drawbacks (at least in the context of my own research field), together with the fact that sometimes, we do not even understand what is going on in the black box. In this way, other methods that are possibly less powerful could be preferable (as we have more control).
I'm glad you found it interesting enough :).. I know we'll continue to develop methods and tools to help us have a better grasp of what goes on in the black box! Plus every drawback opens the door for new research. Who knows what we could stumble upon 😃?
I ask this to myself, every single day ;)